Unleashing the Power of Image Labeling Tools in Business
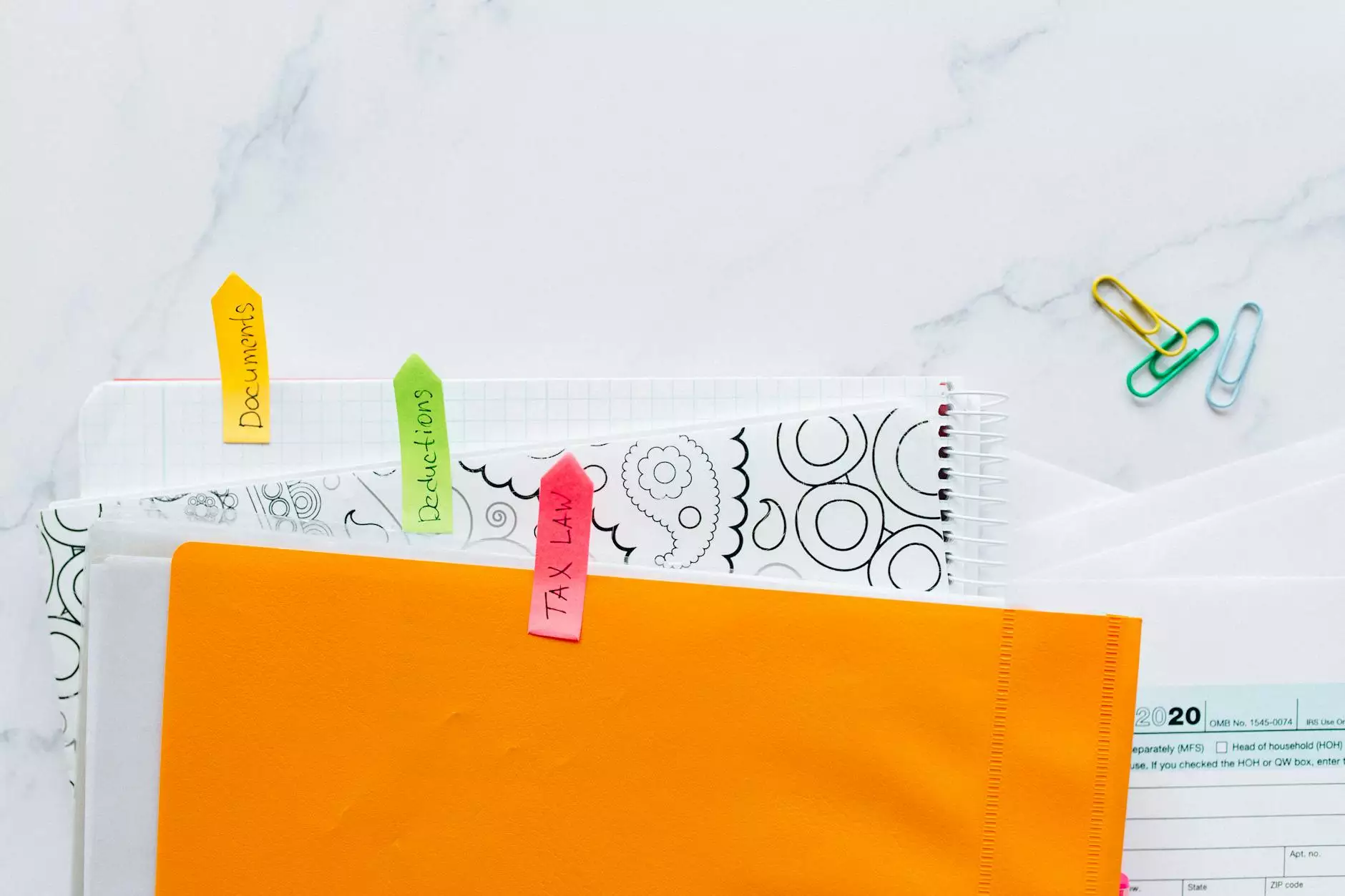
In today's rapidly evolving digital landscape, businesses are facing an unprecedented amount of data. With the rise of artificial intelligence and machine learning, the need for effective data annotation has never been more critical. One essential component of this process is the image labeling tool, which is pivotal for training algorithms and enhancing the accuracy of AI models. In this article, we will explore the myriad benefits of image labeling tools, their role in data annotation, and how they can propel businesses to new heights.
Understanding Image Labeling Tools
An image labeling tool is a software application that allows users to annotate images by tagging objects, identifying categories, and providing metadata. This process is crucial for machine learning, as algorithms require labeled data to learn and make predictions accurately. The effectiveness of a machine learning model is directly proportional to the quality and quantity of labeled data it trains on.
Key Features of Image Labeling Tools
- User-Friendly Interface: Modern image labeling tools typically feature intuitive interfaces that allow users to label images efficiently without significant technical expertise.
- Multi-Format Support: These tools often support various image formats, ensuring versatility for different projects.
- Collaboration Features: Many platforms offer features allowing teams to collaborate in real-time, enhancing productivity and consistency in labeling tasks.
- Quality Control Mechanisms: Built-in features help maintain labeling accuracy through automated checks and team reviews.
- Export Options: Users can easily export labeled data in formats compatible with different machine learning frameworks.
The Importance of Data Annotation
Data annotation transforms raw data into valuable insights. By labeling images, businesses can train their AI systems effectively, thereby improving decision-making processes. The significance of image labeling in sectors such as healthcare, automotive, and retail cannot be overstated. For instance, in healthcare, accurately labeled images of medical scans can assist in early disease detection and improve patient outcomes.
Benefits of Using Image Labeling Tools
The implementation of image labeling tools offers numerous benefits to businesses:
- Enhanced Accuracy: Accurate image labeling is essential for the performance of machine learning models. Properly labeled data allows these models to make better predictions and decisions.
- Time Efficiency: Automating the data labeling process reduces the time required to prepare datasets, allowing teams to focus on other essential tasks.
- Cost Effectiveness: By improving labeling efficiency and accuracy, businesses can save on costs associated with errors and rework.
- Scalability: Image labeling tools provide the ability to scale annotation efforts quickly, accommodating growing datasets effectively.
- Adaptability to Various Industries: These tools can be customized to meet the specific requirements of different sectors, ensuring that the labeling process aligns with business goals.
Choosing the Right Image Labeling Tool
Not all image labeling tools are created equal. When selecting a data annotation platform, businesses should consider the following factors:
- Functionality: Assess whether the tool meets your specific labeling needs, including types of annotations required (bounding boxes, segmentation, etc.).
- Integration: Ensure that the tool integrates seamlessly with your existing workflows and systems.
- Support and Training: Look for providers that offer comprehensive support and training resources to maximize the tool’s effectiveness.
- Cost: Consider the total cost of ownership, including subscription fees, training, and potential hidden costs.
- User Feedback: Review testimonials and case studies from other businesses to gauge the tool’s performance in real-world applications.
Best Practices for Effective Image Labeling
To leverage the full potential of image labeling tools, businesses should adhere to best practices in their data annotation processes:
1. Define Clear Guidelines
Establishing clear labeling guidelines is vital to ensure consistency in the annotation process. These guidelines should specify how to label various objects, what categories to use, and any specific requirements unique to the dataset.
2. Collaborate and Review
Encourage collaboration among team members and establish a review process to catch errors and ensure high-quality annotations. Regular feedback loops can significantly enhance the accuracy of labeled data.
3. Utilize Automation Where Possible
Leverage artificial intelligence and machine learning algorithms to automate repetitive labeling tasks. Tools with built-in automation features can expedite the process without compromising quality.
4. Monitor and Evaluate
Regularly monitor the performance of your image labeling efforts. Use metrics to evaluate the accuracy and completeness of the labels and make adjustments as necessary.
Real-World Applications of Image Labeling Tools
The applications of image labeling tools span a wide array of industries, each benefiting from enhanced data annotation:
Healthcare
In healthcare, image labeling assists in diagnosing medical conditions through the analysis of radiological images, such as X-rays and MRIs. Properly annotated images enable AI models to identify anomalies that may indicate diseases, disease progression, or response to treatment.
Automotive
The automotive industry uses image labeling extensively in autonomous vehicle development. Annotating images of road signs, pedestrians, vehicles, and barriers helps train algorithms that enable self-driving cars to navigate safely and efficiently.
Retail
In retail, visual recognition technologies powered by image labeling tools can enhance customer experiences by enabling applications such as visual search, inventory management, and personalized recommendations.
Agriculture
Farmers are utilizing image labeling to analyze crop health through drones and satellite imagery. Accurate labels help identify issues such as pest infestations, nutrient deficiencies, and yield predictions.
Future Trends in Image Labeling Tools
The evolution of technology is paving the way for innovative developments in image labeling tools. Here are emerging trends to watch:
- AI-Powered Annotation: Future tools will increasingly leverage AI to assist in the labeling process, reducing the amount of manual work required.
- Enhanced Collaboration Tools: The rise of remote work will lead to more sophisticated collaboration features, enabling teams from different locations to annotate images in real-time.
- Integration with Other Platforms: Seamless integration with other data processing and visualization tools will be crucial in enhancing the overall data management workflow.
- Focus on Edge Cases: As machine learning applications become more complex, image labeling tools will need to address edge cases and rare events more effectively.
- Sustainability Considerations: More tools will be developed with a focus on minimizing environmental impact, aligning with the global push for sustainability.
Conclusion
In conclusion, the role of image labeling tools in business is monumental in the age of data-driven decision-making. By harnessing the power of these tools, organizations can optimize their data annotation processes and enhance the performance of their AI systems. As technology advances, the capabilities of these tools will continue to expand, paving the way for more efficient, accurate, and innovative applications across various industries. To ensure your business remains competitive and leverages the full potential of machine learning, investing in a reliable and efficient image labeling tool is not just recommended; it’s essential.
For more information on top-tier image labeling tools and data annotation platforms, visit KeyLabs.ai.